Sub-surface, yet-undiscovered cultural heritage sites (such as buried ancient structures and monuments) can be identified on remote sensing data from a variety of sensors in the form of anomalies or traces detectable on bare soils, crops, and vegetation. Current availability of free remote sensing datasets through platforms, like those offered by Copernicus Service, is unprecedented. However, such extraordinary proliferation of data has posed significant hurdles in terms of managing, processing and interpreting images to the point that the quantity of data is not manageable by traditional ‘human’ visual interpretation.
The new challenge in cultural heritage remote sensing scholarship, therefore, is to develop or improve approaches that can facilitate the automatic detection of (archaeological) objects of interest. CLS project takes charge of such a challenge by developing Artificial Intelligence (AI) algorithms that search remote sensing images of specific cultural heritage objects and patterns related to past anthropogenic interference on landscapes. This project will also considerably expand existing means of identification of ancient land division systems —and more generally of landscape patterning— by automating procedures of similarly-oriented linear feature detection.
In pursuit its research agenda, Cultural Landscapes Scanner (CLS) will work toward removing some of the most pressing barriers to the development of mature applications of AI for automated detection in archaeology, such as:
- lack of suitable training datasets, often limited in span and/or quality and not publicly available;
- absence of publicly available benchmark datasets against which different Artificial Intelligence techniques can be tested;
- lack of a standard set of measures to assess the performance of the different methods.
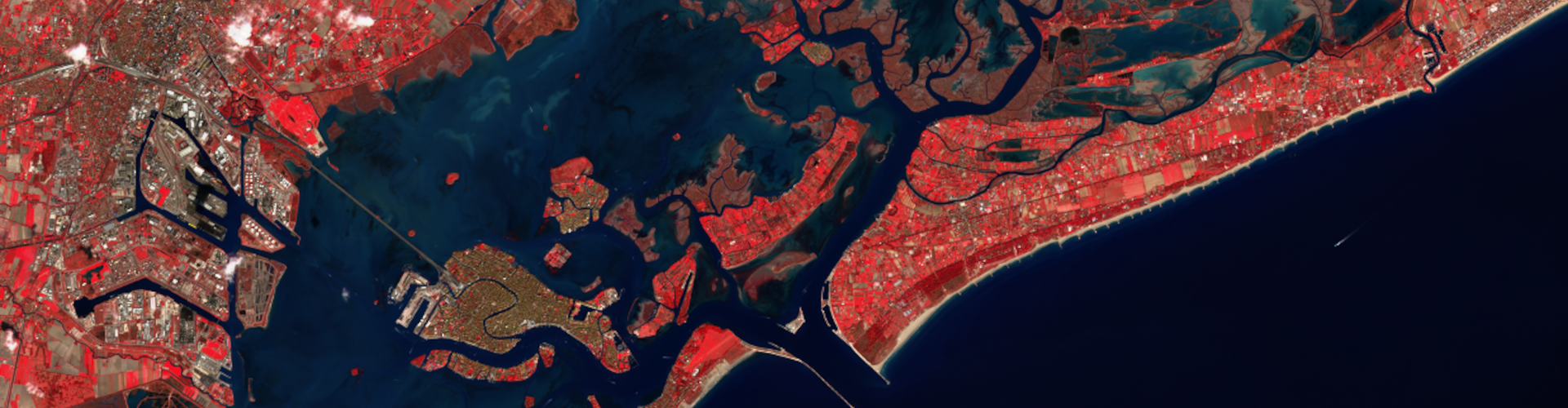